ChatGPT is dominating today's headlines. And for good reason. The latest product release from Open AI and the technology it’s based on, generative AI, has the potential to disrupt entire industries. But how will this new technology affect contact centers??
In this article, we'll tackle everything contact center leaders need to know about generative AI.
What is Generative AI?
Generative AI is a type of artificial intelligence that can generate new content, such as text, images, and audio. It does this by using algorithms to learn from data and then generate new examples that are similar to the training data.

Generative AI has been around for a long time, introduced in the 1960s in chatbots. Generative AI builds on Large Language Model (LLM) related technology that has accelerated in the last few years. The recent advancements in generative AI and LLM technology has opened the floodgates in terms of the applications that can be created across many different verticals, including the contact center industry.
What is a Large Language Model (LLM) and how does it work ?
A large language model is trained to understand and generate human language. LLMs have a much larger vocabulary and a greater capacity for understanding complex language structures, nuances, and context than language models.

There are many LLMs that have been developed over the years. Each is trained on a different size of parameters as shown above. Apart from architectural innovation, the two main driving forces have been larger data sets and larger model complexity (i.e. # parameters). For example BERT was trained on ~3.3B words while GPT-3 was trained on ~45T words (~15000 times larger) and GPT-4 could be orders of magnitude higher. Moreover, the ecosystem of open and closed source models are growing every day at a rapid pace providing more avenues for innovation.
What contact center leaders should know about Generative AI
ChatGPT, which is based on generative AI, is all the buzz right now and contact center technology companies are eager to jump on the opportunity to appear innovative and provide generative AI-powered products. However, before you jump in, you should be aware of both the advantages and disadvantages of generative AI technology, using different LLMs.
Generative AI applications built on top of advanced NLP technology will enable the application to understand and interpret natural language input from users. Generative AI can be used to build specialized applications focused on improving contact center interactions like call summaries, conversation insights or knowledge AI to help agents in real time. It is important while building these applications that care must be taken to handle the noisiness of contact center data by fine tuning the models.
One of the biggest limitations is that the AI will need to adapt to each company’s data, in this case transcripts of customer conversations. AI is not sophisticated enough to understand with 100% accuracy which agent responses were the best to use as knowledge assets for other agents. The AI cannot discern and learn only from the right or best responses. The AI can often make up information and can provide completely inaccurate or irrelevant responses. If an agent uses an incorrect AI output in their response to a customer, it can have a serious impact on customer experience and compliance. AI vendors need to spend time analyzing these mistakes and vetting the viability of Generative Pre-trained Transformer (GPT)models in a business setting.
Additionally, it is a very challenging task to train LLM for spoken language text (e.g contact center data, where natural conversations happen between the customer and the agent) and noisy data, where errors are prevalent in the transcribed text. These errors significantly degrade the performance of downstream tasks.
How Observe.AI uses Generative AI for conversation intelligence
At Observe.AI, we have been building large language models with an emphasis on contact center interactions since the beginning to understand and analyze customer conversations for companies like Public Storage, Bill.com, Accolade, Cox Automotive, and more. By using trained LLMs specific to contact center data and designed for key classification use cases, such as sentiment analysis, dead-air detection, entity detection, speaker diarization and recognition, language identification, etc, we are able to deliver a deeper understanding of contact center customer interactions beyond what can be delivered using generic LLMs.
For example, to address the noisy transcription problem, we have built a model that leverages phoneme sequences as complementary features, making it robust to transcription errors. Our experiments indicated that this approach is able to recover up to 90% loss in performance of out-of-box LLMs occurring due to noisy transcripts. We encode all these properties of spoken language into LLM using a multi-task learning framework so that the model learns them together. Thus, these LLMs help in building supervised task-specific models for various contact center tasks such as sentiment classification, dead-air identification, entity recognition, with very high accuracy, fewer training examples and smaller models.
With a large language model purpose-built for the contact center, we’re able to get more accurate results for tasks such as dead-air, sentiment and entity detection that are anywhere between 1.1 to 1.5x more accurate compared to GPT. These generative AI models are not only optimized for the contact center domain but also provide a higher level of trust, and control than generic open-source or 3rd party LLMs (which are the technologies behind GPT and ChatGPT).
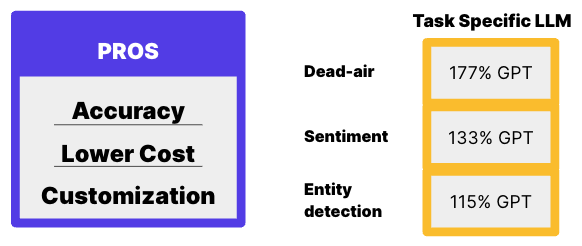
Improved Customer Experience Through Task Specific LLMs
There is a massive opportunity for contact centers to begin experimenting and innovating with GPT-family and other LLMs. Call summarization, knowledge AI, conversation insights, coaching AI are just a few of the applications and use cases for this new innovation.
GPT models will continue to be helpful in building quick prototypes, testing hypotheses and accelerating innovation, augmenting large language models that are built specifically for the contact center. This approach offers more control and trust over the model as well as feedback loop to improve the mode and fine-tune it to the needs of every business.
As the world watches what’s next for ChatGPT and generative AI, we’ll be working behind the scenes to bring you game-changing innovation so you can drive better business outcomes across your contact center.