NAACL, the North America chapter of the Association for Computational Linguistics (ACL), is one of the foremost conferences in the field of Natural Language Processing (NLP). This year, the conference was held in the vibrant and culturally rich city of Mexico City. The event was a grand convergence of leading minds in NLP from academia and industry, featuring the presentation of over 1580 papers that spanned a wide array of themes and tracks, including the plenary talks and keynotes on Harnessing the Power of LLMs to Vitalize Indigenous Languages and Distributional Semantics: What do large language models have to say?. The accepted works varied from the topics such as the training and evaluation of large language models (LLMs), dialog systems, question-answering frameworks, knowledge base construction and retrieval mechanisms, to the study on safety, harmfulness and bias of the LLMs and innovative multimodal architectures, among others. The diversity and depth of the research presented underscored the dynamic and rapidly evolving landscape of NLP, and we are proud to have contributed to this vibrant scientific discourse.
At Observe.AI, we develop contact center Large Language Models (LLMs) that are specifically designed to enhance the performance of downstream tasks in the contact center industry. Observe.AI Contact Center LLM, is meticulously trained on real-world contact center data, enabling it to deliver better accuracy, control, and privacy for contact center specific workflows.. Specifically, our Contact Center LLM is 35% more accurate on the task of summarization compared to GPT3.5, and 11% better compared to GPT4. Our domain-specific LLM stands apart from generic models by focusing on the unique challenges and requirements of contact centers. This specialization allows for superior performance in tasks such as real-time question answering, automated call summarization, and generating coaching notes for agents, all while maintaining stringent privacy standards.
In pursuit of developing the Observe.AI Contact Center LLM, we submitted some of our findings to the NAACL 2024 conference. These research papers highlight our team's past and ongoing work in the field of training and evaluating LLMs in the contact center space, and are instrumental to bringing more value to generative AI use cases for our customers.
- Tweak to Trust: Evaluating the reliability of summarization metrics in contact centers via perturbed summaries.
- The Paradox of Preference: Examining the impact of data collection methods on LLM performance and alignment algorithms.
- Probing Classifiers: Investigating how conventional probing tasks fail to capture the nuanced learning of contact center-tuned LLMs.

Paper Descriptions
Tweak to Trust: Evaluating the Reliability of Summarization Metrics in Contact Centers [Ref: https://aclanthology.org/2024.trustnlp-1.14.pdf]

In this paper, our team assessed the robustness of summarization metrics by introducing systematic perturbations to summaries in contact center datasets. Inspired by the analysis of patterns of errors of the summarization model during our error analysis, we introduce domain specific perturbations to test the reliability of the existing out-of-the-box summarization evaluation metrics. We noticed that perturbing the output resulted in upto 12-15% degradation in correlation of evaluation scores such as traditional metrics like Rouge, Bleu, as well as recent evaluation metrics of BERTScore and Unieval scores, to human evaluation. This variance highlights the instability of these metrics in practical settings, suggesting they may not reliably reflect true summarization quality in real life contact center calls. Our findings advocate for the development of more robust and domain-specific metrics that can account for the complexities of real-world data. This finding has been crucial to setup an in-house monitoring system to evaluate GenAI capabilities such as summarization [Ref: https://www.observe.ai/blog/evaluating-llms-in-contact-centers]
The Paradox of Preference: Examining the Impact of Data Collection Methods on LLM Performance and Alignment Algorithms [Ref: https://aclanthology.org/2024.insights-1.16.pdf]

This study investigated the effects of different data collection methodologies on the performance of alignment algorithms, including Direct Preference Optimization (DPO), Identity Preference Optimization (IPO), and Conservative DPO (cDPO). Notably, our findings revealed that DPO models trained on LLM-generated preferences performed approximately 15% better on alignment tasks than those trained on human-annotated preferences, contrary to expectation of IPO to demonstrate the improvements over DPO, as shown in the IPO paper. Additionally, we observed no significant correlation between the volume of preference data and performance improvements, challenging the assumption that more data necessarily leads to better model alignment. We have been utilizing such findings to steer the development and iterations of our own Contact Center LLMs. [Ref: https://www.observe.ai/press-releases/contact-center-llm-generative-ai]
Probing Classifiers: Investigating Why Conventional Probing Tasks Fail to Capture the Nuanced Learning of Contact Center-Tuned LLMs [Ref: https://aclanthology.org/2024.insights-1.12.pdf]
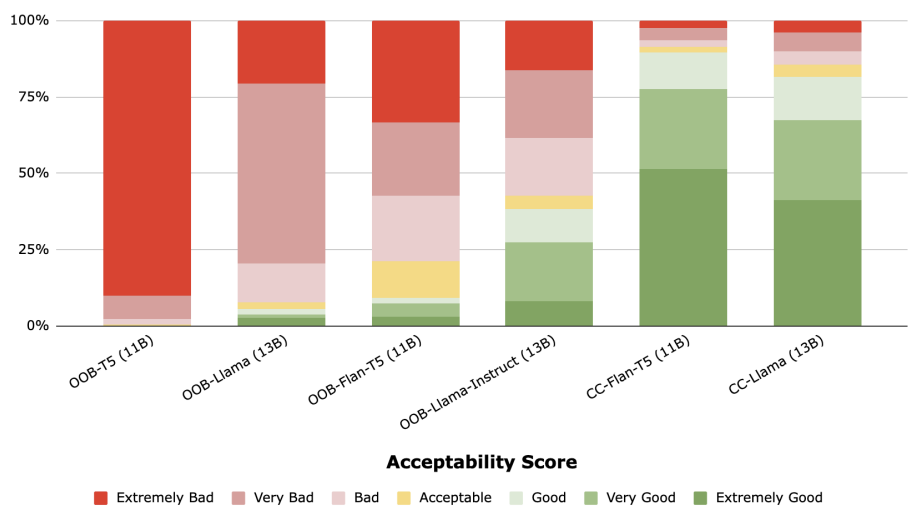
In an effort to identify a low-resource setting to evaluate the domain specific understanding of the LLMs, our research focused on the efficacy of traditional probing tasks in evaluating the capabilities of LLMs fine-tuned for the contact center domain. Despite a 48% improvement in downstream task performance metrics, such as call summarization and sentiment analysis, the probing tasks did not reflect this improvement. For instance, in tasks assessing linguistic nuances like disfluency detection and conversational turn-taking, the probing results showed minimal differentiation between fine-tuned and baseline models. This discrepancy highlights the need for developing specialized probing techniques that can more accurately measure the unique competencies of domain-adapted LLMs.